Understanding Large Quantitative Models
Explore the significance and applications of large quantitative models in various industries, enhancing decision making and strategic planning through data driven insights and advanced analytics in this AI World
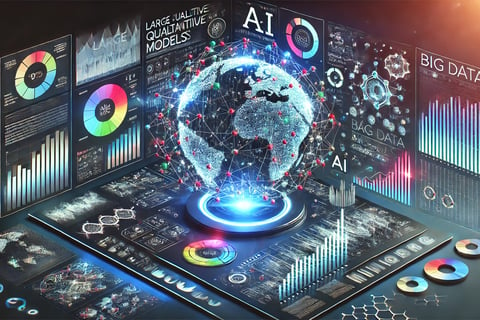
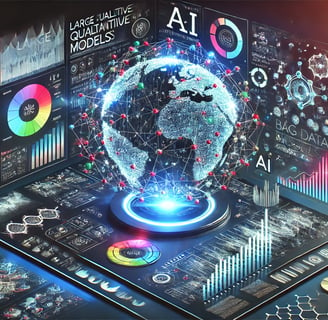
Large Quantitative Models
Explore the importance, applications, and benefits of large quantitative models in various industries.
What is LQM (Large Quantitative Models) ?
Large Quantitative Models (LQMs) are advanced computational models designed to process vast datasets and perform complex quantitative analyses. These models leverage sophisticated algorithms and extensive computational resources to analyze numerical data, detect patterns, and make predictions or decisions based on statistical and mathematical principles.
In the context of AI, LQMs often utilize techniques such as machine learning (ML), deep learning, and reinforcement learning to build predictive or decision making systems. Unlike qualitative models, which focus on subjective and descriptive data, LQMs handle numerical and structured data, enabling precise calculations and forecasts.
How Large Quantitative Models Are Used in AI
AI systems use LQMs to analyze trends and patterns in large datasets. For example, in financial markets, models analyze historical price data to predict stock movements.
Data Analysis and Prediction
Optimization
Logistics and supply chain management use LQMs to optimize routes, inventory levels, and resource allocation, reducing costs and improving efficiency.
Simulation
Models simulate real world systems, such as traffic flow or climate conditions, providing valuable insights for decision making.
Risk Assessment
In industries like insurance and finance, LQMs assess risks by analyzing probabilities and potential impacts of events.
Machine Learning
Training large scale ML models (e.g., neural networks) often relies on LQMs for gradient calculations and hyperparameter tuning